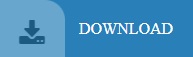

Many autonomous vehicles require precise local-ization into a prior map in order to support planning and to leverage semantic information within those maps (e.g. We processed almost a billion points spanning an area of 3.3 km2 in less than an hour on a regular desktop. The methods have been applied to areas spanning several kilometers in multiple cities with data collected from both aerial and ground sensors exhibiting different properties. Our algorithms work across a large spectrum of urban objects ranging from buildings and forested areas to cars and other small street side objects.
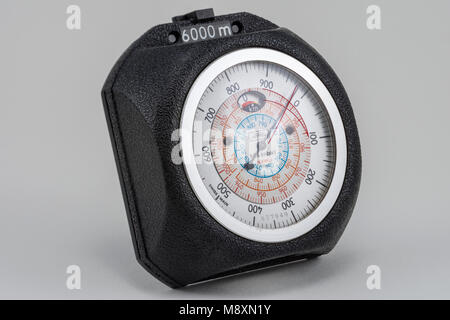
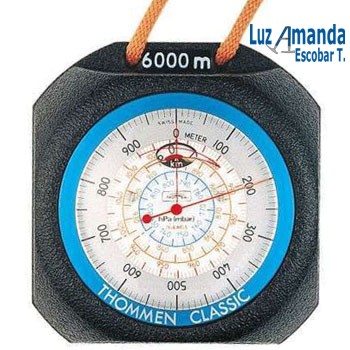
This scheme captures the nature of the real world, thereby making segmentation tasks intuitive and efficient. A key contribution is our novel Strip Histogram Grid representation that encodes the scene as a grid of vertical 3D population histograms rising up from the locally detected ground. Segmentation is crucial because it allows further tasks such as recognition, navigation, and data compression to exploit contextual information. As part of a large-scale 3D recognition system for LIDAR data from urban scenes, we describe an approach for segmenting millions of points into coherent regions that ideally belong to a single real-world object.
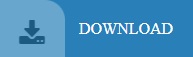